- 19.1k views
Clinical AI Prediction Tools: Opportunities, Barriers, and the Road to Adoption
Clinical AI prediction tools offer significant opportunities to enhance patient care by providing early insights into potential health risks, improving diagnostic accuracy, and enabling personalized treatment plans. These tools can analyze large datasets, including medical history, genetic information, and real-time patient data, to predict outcomes such as disease progression or response to treatment. However, challenges such as data privacy concerns, the need for high-quality datasets, and integration with existing healthcare systems pose barriers to widespread adoption. Additionally, regulatory hurdles and ensuring clinician trust in AI-driven recommendations are critical factors for successful implementation. Overcoming these challenges requires collaboration between healthcare providers, AI developers, and regulatory bodies to ensure these tools are both effective and safe.
About the Speaker
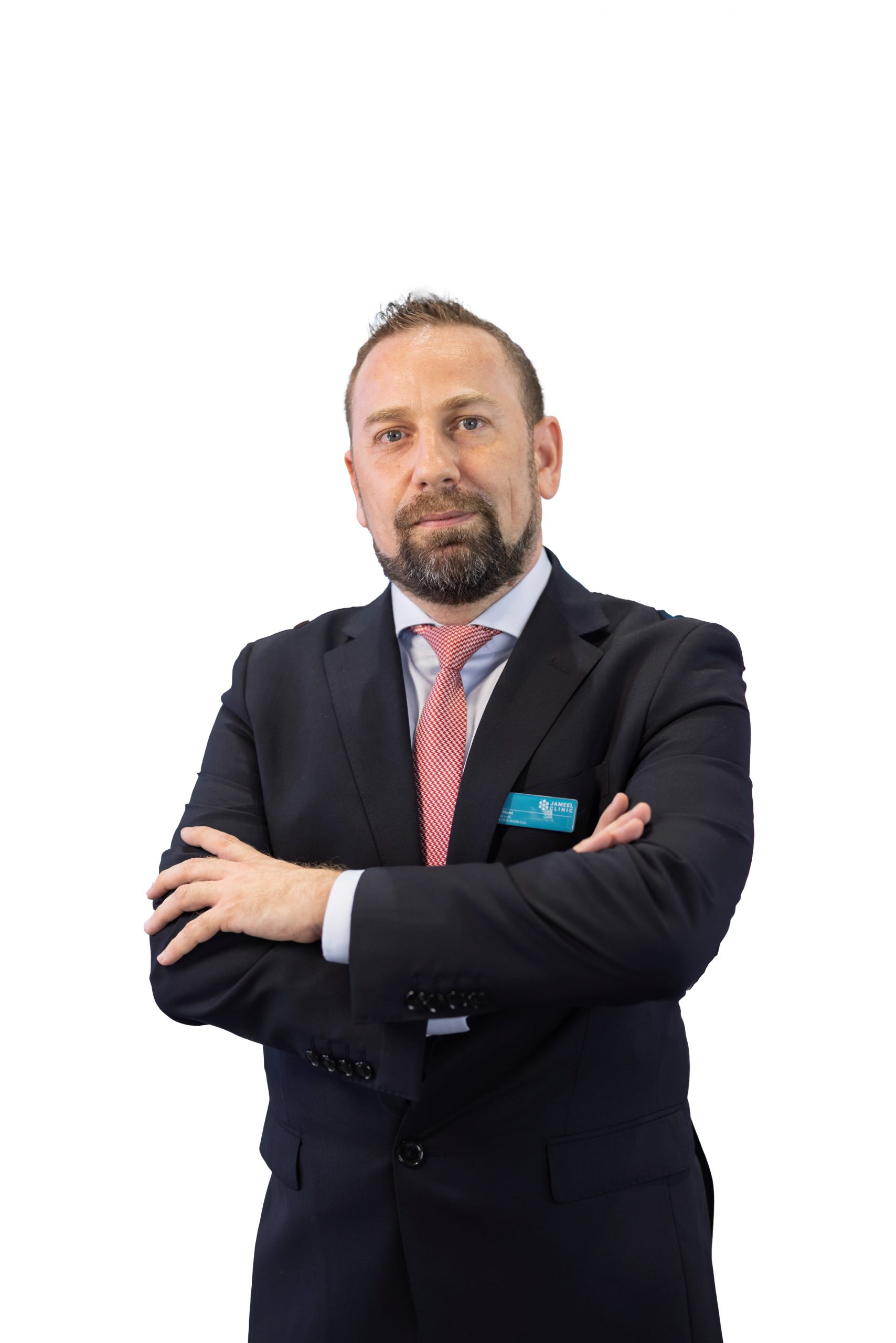
Dr. Nacer Mami
Regional Lead Clinical Network, MIT Jameel Clinic, Dubai
Dr. Nacer Mami, Regional Lead Clinical Network, MIT Jameel Clinic, Dubai
Upcoming Case Discussions
Preparing your Practice for Adoption of AI
Preparing Your Practice for Adoption of AI" involves building a foundation of digital literacy, data readiness, and ethical awareness among clinicians and staff. Successful integration starts with identifying key workflows where AI can enhance efficiency, accuracy, or patient outcomes. It’s essential to choose tools that align with your practice’s goals while ensuring transparency, security, and compliance. Training, change management, and continuous evaluation are critical to fostering trust and long-term adoption. Ultimately, AI should support—not replace—the human touch in medicine.
Inspiratory Muscle Training: Benefits & Techniques
Inspiratory Muscle Training (IMT) is a targeted exercise program that strengthens the muscles involved in breathing, particularly the diaphragm and intercostal muscles. It is beneficial for individuals with respiratory conditions such as COPD, asthma, and heart failure, improving lung function, reducing breathlessness, and enhancing exercise tolerance. IMT can also benefit athletes by increasing endurance and respiratory efficiency. Techniques involve the use of handheld devices that provide resistance during inhalation, with training typically performed twice daily for several weeks. Consistent practice leads to better respiratory muscle strength, improved quality of life, and greater overall physical performance in both clinical and athletic settings.
Rickets & Vitamin D Deficiency: Prevention & Treatment
Rickets, caused primarily by vitamin D deficiency, leads to impaired bone mineralization in children, resulting in bone deformities, delayed growth, and skeletal pain. Prevention involves adequate sunlight exposure, a diet rich in vitamin D and calcium, and supplementation in at-risk populations such as exclusively breastfed infants or those with limited sun exposure. Treatment includes vitamin D and calcium supplementation, correction of underlying causes, and monitoring of biochemical markers and bone health. In severe cases, high-dose vitamin D therapy may be required. Early diagnosis and intervention are crucial to prevent long-term complications and support healthy growth and development in children.
Sleep Apnea and Daytime Fatigue
Sleep Disorder Breathing is a term for a group of conditions with abnormal breathing patterns during sleep. This affects everyday functioning and well being.There are few types of SDB which include upper airways resistance, hypopnea, apnea, catathrenia and heavy snoring. People suffering with SDB can have daytime symptoms that result in poor sleep also. There can also be sleepiness, depressed mood, irritability and cognitive dysfunction.
Common Surgical Emergencies in Newborn – Case scenarios
Neonatal surgery is an emergency service; it would not be appropriate to use extremely expensive equipment and highly specialized skills for a procedure that might wait. In fact, most neonatal surgery is performed with the intention of preserving lives. New surgical techniques for the treatment of other congenital anomalies are improbable, except from the inevitable rise in operations for congenital heart disease during the newborn period. The majority of specialized neonatal surgical units have found that children do not pass away from the condition for which they were referred; instead, they pass away from severe prematurity, respiratory difficulties, and accompanying abnormalities, many of which are incurable. Any improvement in outcomes is probably going to come from ventilation.